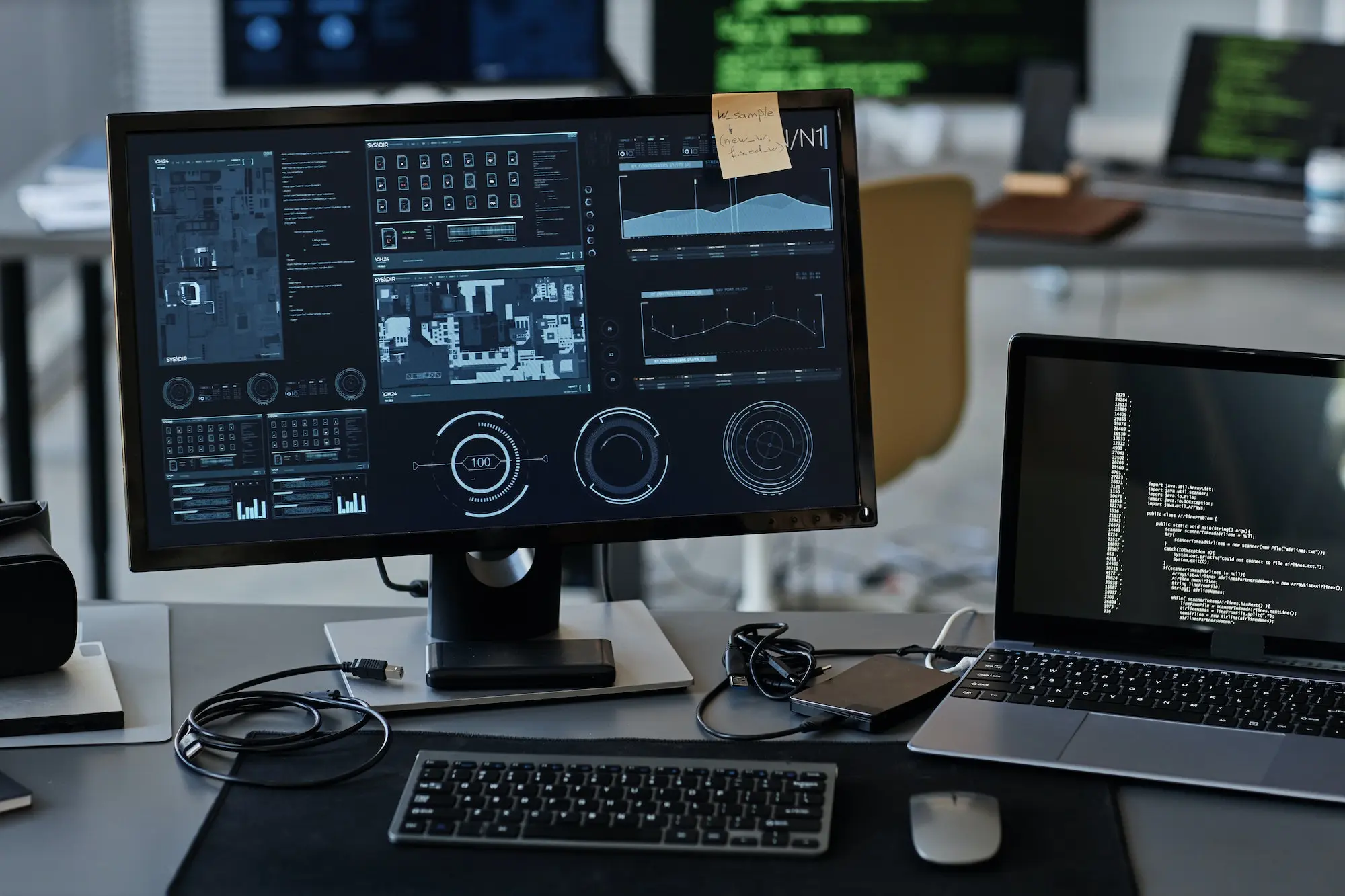
Data science is a composite of mathematics, statistics, and computer science. It is used to assist in decision-making and strategic planning, thus meaningful data leads to more accurate predictions. Millions and millions of data are gathered, and, through data science, highly accurate predictions are made. Data scientists use this to predict outcomes in minutes, which would take several days to years for a human to process. Most of the technology that we use nowadays makes use of data science. If you open your phone right now and go into the weather app, you will be able to see all those weathers and temperatures for a week ahead. YouTube video suggestions, Netflix series and movie suggestions, Google search suggestions and advertisements; data science has a role in all of these. They gather data on previous weather, video history, and relevant historical data, and make a prediction out of it. Data science can be also used to determine what an image is showing, recognize faces, read out any languages, and so on. There are many types of data science of applications.
Prediction models
Prediction models are used to make predictions about future events and outcomes. The model gathers data from the past, analysis it, and makes predictions based on the analysis.
By making use of prediction models, doctors can determine conditions much quicker and easier. It can also be used to keep track of the patient’s health indicators and predict any possible disorders. This was all possible by the gathering of previous patient medical records, medical exam results, and all the necessary data. Then by using data science, the predictions are made. The predictions are not always correct, so it is in the doctor’s hands to make the final decision.
Prediction models are also commonly used in fields such as finance, marketing, and more. A prediction model can be used to predict stock markets, customer behavior, market risks, and so on. Business companies benefit from these prediction models by using them for analysis of their company.
Internet search and target advertising
When searching through the internet, especially Google, you will often see a list of autocompleted questions. This is done through data science. The record of what people search gets stored and by the frequency of similar searches, it recommends a suitable search recommendation for you.
Google also displays adverts based on this. They keep track of your searches and show you the most relevant adverts. If you choose a topic and search about it for a bit, Google will start displaying adverts related to it.
This is all done through cookies. Often websites will ask you to allow them to drop a cookie. Not all websites do this since this is based on where their site is made and the law in that country. A cookie is a friendly word for a tracker. You will most likely say no if they asked you to allow them to drop a tracker, but a cookie is much more friendly. By them dropping a cookie (tracker) they keep a track of all your activities on the website. This then gets sold to the vendors under their list. This is how user data gets extracted.
Image recognition
Image recognition is broadly used in most applications. iPhone has a Face ID feature where it allows you to unlock your phone using your face. We don’t always have the identical look every day, but surprisingly, Face ID works pretty well. We sometimes gain weight, lose weight, put on make-up, but Face ID will most likely recognize you.
When you post an image that you took with your friends on Facebook, it suggests tagging your friends and this is also fairly accurate. This works through Facebook keeping a record of all users’ profile pictures and going through image recognition.
That horrible reCAPTCHA that you have to go through when you sign in or register also uses image recognition. The system knows what it is asking, and which images should be selected. The ironic part is that a robot is checking whether a person registering is a robot or not.
Unfortunately, face recognition is not always used for good. It can be used to make videos of imitating others. This is called deepfake. Deepfake is used to replace a face of a person in a video with the face of someone else. When deepfake first came out it was barely usable, and you could clearly see that the video has been edited. Nowadays, deepfake got much better and it is really difficult for a human to determine whether it is real or not. Deepfake can be used to make fake news or to cyberbully. Not every feature of data science is beneficial.
Natural Language Processing (NLP)
Natural Language Processing is a study of the interaction between computers and humans in human language. This involves speech recognition, machine translation, analysis of sentiment, etc.
One of the most used applications that involve NLP is a machine translation. With the use of machine translation, the translation of languages can be done within a minute. NLP is used in Google translate by automatically translating one language into another one. Some languages use alphabets, whereas other language uses symbols. Different languages also have different grammar. Machine translation is not always accurate due to the ambiguity and most languages having their own metaphors and so on. People are sometimes ambiguous even when they communicate in their mother tongue. The advance in modern data science made it possible to build applications that work with human language.
Speech recognition is also a part of NLP. This is used for processing spoken words into text. People have different accents, and people sometimes struggle to understand others with accents that they find difficult to understand. NLP makes this process easier and faster, but, as mentioned above, it is not always accurate. NLP it is getting better and better as data science is improving. Computers translate spoken words into text and then they process it. This is how applications like Siri and Google voice work.
Data mining
Data mining involves pattern recognition and data inspection. Data mining can be used to group customers based on their behavior such as their purchase history. This is then used to identify products that are purchased regularly. The results are used for improving market strategies and improving customers’ experiences when shopping.
It is essential to work with mass data, but also meaningful data. When gathering data, it will not always be useful due to errors and outliers. Data mining can be used to clean up these unnecessary data, keeping the data meaningful and reliable.
Automation
Automation is used to perform tasks that were previously done by humans. This can be used to speed up the process by letting the computers do repetitive tasks. By letting the computers do the job, it will increase speed, accuracy, and efficiency. An increase in accuracy leads to fewer errors, which leads to fewer costs. An increase in speed means more productivity. By using an automated system, we increase productivity and decrease costs. Dangerous tasks can also be handled by machines, and the work will be done much quicker due to not having to be cautious.
Automation sounds great, but there are downsides also. Automated systems are not always designed to handle exceptions. For example, an automated machine in an assembly line might cause an issue due to unexpected situations, such as the parts for the assembly not being in the correct direction. The machine might not even stop after a problem has occurred and keep running, which could potentially lead to an accident. It is required for an inspector to always be present to handle those exceptions.
In the current era, with a lack of job opportunities, people will start losing their jobs due to machines doing their job. People will either have to look for a new job or work at a cheaper rate. This could potentially lead to wider social inequality.
Conclusion
Data science has been used broadly in the past, and it is still used in modern days. It is so useful and powerful that it became a key essential in our life. It is true that data science has made our lives better, but as the technology of data science improves day to day, more people will be using it and we will be dependent on it. It is possible that eventually, we will not be able to process our minds due to being too reliant on data science. We will just rely on data science believing that it will make the correct decisions for us, not having a single suspicion. The better data science gets, the more cautious we have to be not to rely on it too much. Whether it is data science or money, anything that makes your life more luxurious, we must control it and not let it control us.